Commercial Excellence - Elevate Your Lead to Order Process with Predictive Analytics!
- dtotte
- Jan 15, 2023
- 2 min read
Using predictive analytics within the lead to order process is becoming increasingly popular as businesses look for ways to drive commercial excellence. By following these 7 steps, companies can effectively collect, analyse and use data to effectively inform the decisions that could make all the difference when it comes to improving sales performance.
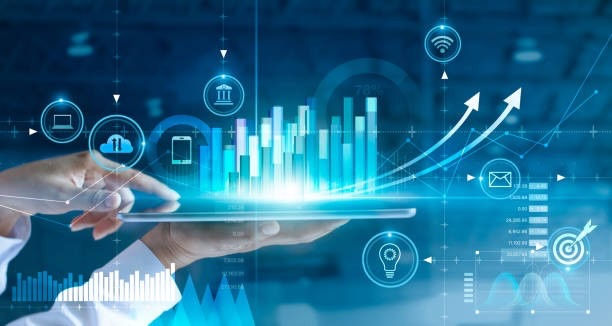
1. Create an Opportunity Pipeline. The first step to building a predictive analytics solution focused on the lead to order process is to create an opportunity pipeline, which is a comprehensive view of sales opportunities and how they relate to the pipeline stages. This pipeline should include all the stages necessary to move a lead from being identified to signing the order, such as Qualification, Proposal, Negotiation, and Finalisation.
2. Data Collection. Once the sales opportunity pipeline is in place, the next step involves collecting all the necessary data for each stage and opportunity. Depending on the complexity of the sales process, this could include customer information, contact details, order size, product details and requirements, etc. This data should be stored in a database or a CRM system in order to be accessed later.
3. Data Wrangling. Before being able to use the collected data for predictive analysis, it is necessary to prepare the data by cleaning, transforming, and formatting. This includes ensuring that the data adhere to the analytic models’ requirements, that all unnecessary data are deleted and all relevant data are available. In addition, variables might need to be coded and record linkage may be necessary.
4. Model Building. After prepping the data, the next step is building predictive models. The models should be tailored to the questions specific to the sales opportunity pipeline in order to find useful insights.
5. Model Evaluation. The predictive models should be evaluated against the appropriate metric to determine its accuracy. Depending on the complexity of the problem and the desired accuracy, traditional statistical methods or machine learning algorithms might need to be used.
6. Model Deployment. Once the models have been validated, it is necessary to deploy them in the production environment. This includes connecting them to the database or CRM system, as well as deploying automation tools to ensure that the model gets regularly re-calibrated and updated with the most recent data.
7. Results Monitoring. The final step is to track and monitor the model's performance to ensure that it continuously delivers desired output. This includes measuring the accuracy of the model and taking corrective steps when needed.
At DECISUS, we can help you start your journey in analytics and data science. Whether you're just starting out in the field or looking to build on your existing analytics platform, our team of expert professionals can provide guidance, advice and the know-how to help you reach your goals. Contact us today to learn more.
Comentarios